
In these small areas, Gray Level Co-occurrence Matrix (GLCM) was computed, which aggregated the distribution of co-occurring values. To reduce the computational complexity, the algorithm randomly selected relatively small areas that correspond to the same fragments among digital images of the same slide. The resulting digital slides were manually registered to compensate for the constant translation.
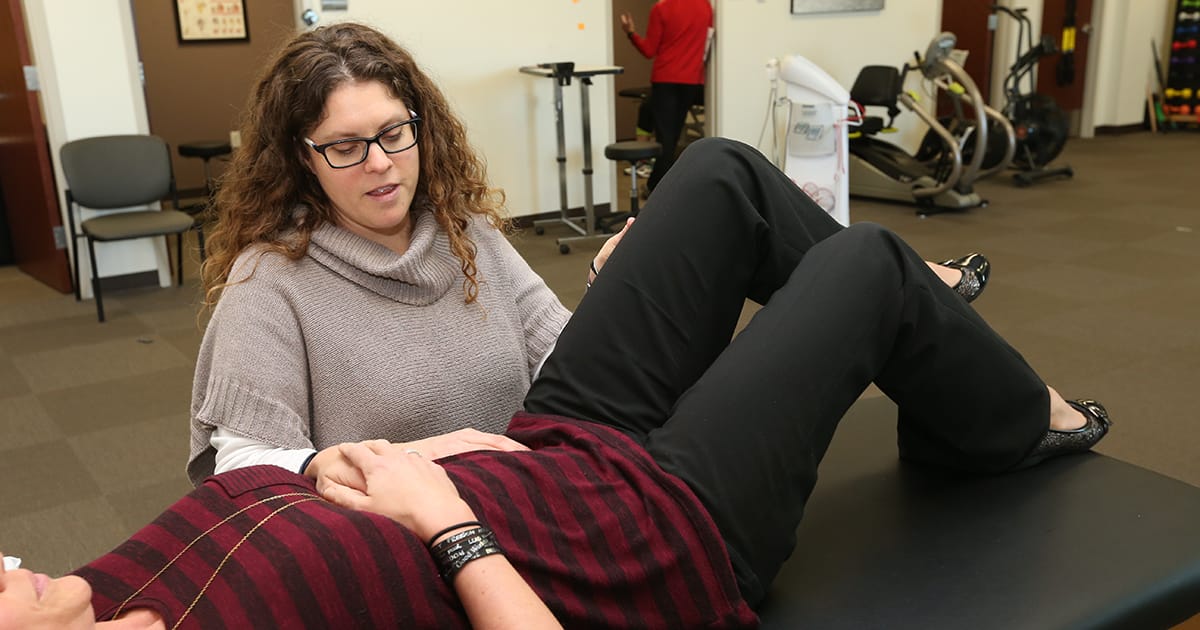
They scanned the same glass slide with multiple scanners and noticed that each scan resulted in a constant amount of translation in the planer space, which made it difficult to fairly compare among digital slides. Walkowski and Szymas developed an algorithm to compare the quality of digital slides generated by different scanners. In recent years, a few systems have been developed to identify scanning artifacts automatically from digital slides. It also disrupts the workflow as it will be easy to miss blurry regions until the time of clinical review, which will delay the diagnosis of the case. Currently, blurry regions are identified manually, a process that is subjective, tedious, error-prone, and time-consuming. If the amount of blurring is minimal (acceptable levels are to be determined in advance), the resulting images can still be presented to pathologists or algorithms. If these areas can be identified, the slides can be rescanned with additional focus points in areas of blurring. When parts of an image are blurry, this affects the performance of both pathologists and automated image analysis algorithms. the larger the distance, the more blurriness it will result. The distance of the focus points from the actual tissue plane is proportional to the amount of blurriness, i.e. However, commercial scanners may still produce digital images with out-of-focus/blurry areas if their AF optics system erroneously selects focus points that lie in a different plane than the proper height of the tissue.

From these focal planes, scanners capture images to produce sharp tissue representation. AF optics systems determine a set of focus points at different focal planes to be perfectly aligned with tissue height that may slightly vary within a slide. Most modern scanners come equipped with autofocus (AF) optics system to select focal planes to accurately capture three-dimensional tissue morphology as the best two-dimensional digital image. Along with spatial resolution and color depth, image sharpness is often used to gauge the quality of digital slides.

High-quality digital slides are becoming a ubiquitous and indispensable clinical workflow and research in pathology. DeepFocus has the potential to be integrated with whole slide scanners to automatically re-scan problematic areas, hence improving the overall image quality for pathologists and image analysis algorithms. When trained and tested on two independent datasets, DeepFocus resulted in an average accuracy of 93.2% (± 9.6%), which is a 23.8% improvement over an existing method.

DeepFocus was trained by using 16 different H&E and IHC-stained slides that were systematically scanned on nine different focal planes, generating 216,000 samples with varying amounts of blurriness. DeepFocus is built on TensorFlow, an open source library that exploits data flow graphs for efficient numerical computation.
Deepfocus careers software#
The aim of this study is to develop a deep learning based software called, DeepFocus, which can automatically detect and segment blurry areas in digital whole slide images to address these problems. Moreover, this process is both tedious, and time-consuming. These areas are typically identified by visual inspection, which leads to a subjective evaluation causing high intra- and inter-observer variability. Moreover, these artifacts hamper the performance of computerized image analysis systems. Unfortunately, whole slide scanners often produce images with out-of-focus/blurry areas that limit the amount of tissue available for a pathologist to make accurate diagnosis/prognosis. The development of whole slide scanners has revolutionized the field of digital pathology.
